In the last two decades weather forecasts have become much more skillful and reliable: Today's 3-day forecasts are about as accurate as the one-day forecasts used to be 20 years ago.
Our department is at the forefront of research that improves prediction via enhanced data assimilation schemes and computing algorithms.
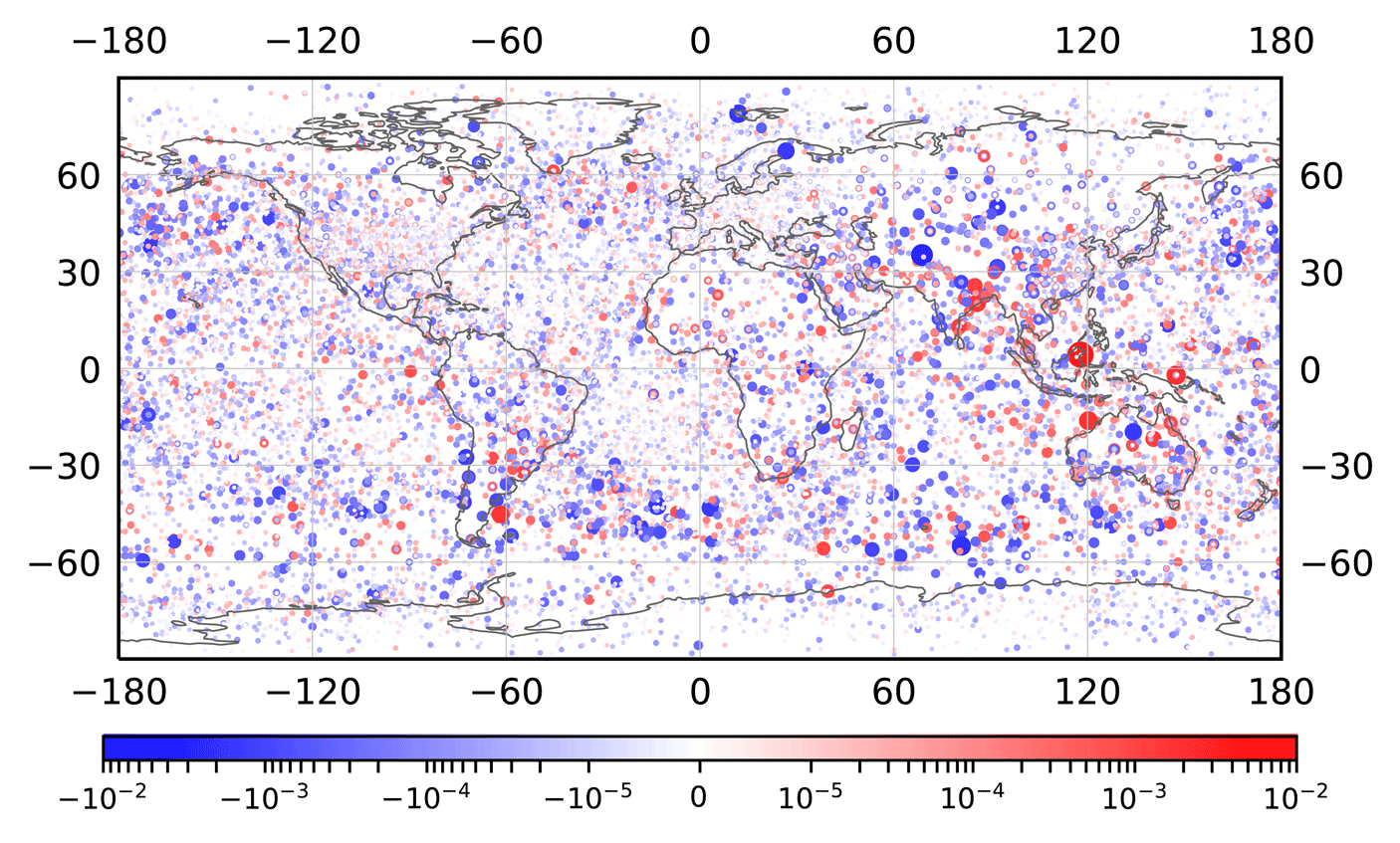
Geographical distribution of conventional observations (NCEP prepBUFR) over a month and how much they improved or degraded short-term forecasts, estimated efficiently using ensemble forecast sensitivity to observations (EFSO). Red (Blue) represent detrimental (beneficial) observations. The magnitude of the EFSO impact is shown by the size of each dot. Proactive Quality Control (PQC) then deletes the most detrimental observations, and the observed improvement of the analysis accumulates with time. Experiments performed with a low-resolution GFS show that if the 50% most detrimental observations are deleted, the skill of the 10-day forecasts increases by more than 12 hours compared to the control. Credit: Tse-Chun Chen and Eugenia Kalnay.
Background
Atmospheric models are based on the physical/dynamical equations that govern the atmospheric flow, written as a computer code. These codes are initialized with data created by combining short model forecasts with new observations (a process called data assimilation). When a model is integrated in time (i.e., the model codes are run) starting from the initial conditions, the output is a numerical weather prediction. These numerical forecasts provide guidance to human forecasters and are the basis of all the National Weather Service and media weather forecasts.
The increase in our prediction capabilities over the past twenty years is mostly attributable to the improvements that have taken place in these computerized weather forecasts, through the better use of the observations, and the use of more advanced models and of more powerful computers.
Our department is involved in many of current developments in Numerical Weather Prediction and Data Assimilation: very high resolution, non-hydrostatic models (mesoscale meteorology) as for example the mesoscale model runs for regional air pollution studies, new numerical methods for the dynamical equations, advanced physical processes, advanced methods for data assimilation, coupled ocean-atmosphere-land forecasts of El Nino, data assimilation for the Atlantic.
See also the UMDWeather-Chaos Project website.
Research Interests
Hugo Berbery has research interests in regional hydroclimate variability, monsoon systems and land surface-atmosphere interactions. He currently serves as co-chair of the International CLIVAR’s Variability of the American Monsoon Systems (VAMOS) Panel and as a member in other international panels.
James Carton collaborates with NASA, NOAA and UMD scientists with the goal of providing consistent analysis of climate variables across air/sea/sea ice boundaries.
Kayo Ide is interested in the dynamics of atmosphere and oceans. Her research in data assimilation focuses on the development of methodology, including Lagrangian data assimilation, observing system design, non-Gaussian filtering, nonlinear filtering. She leads the UMD Weather and Chaos group, which meets monthly and has more than 30 members.
Daryl Kleist research interests include data assimilation, numerical weather prediction, atmospheric predictability, targeted observing, data thinning and forecast sensitivity. His data assimilation research has primarily focused on improving initial conditions through algorithm development for operational numerical weather prediction for short- and medium-range time scales. Most recently, he has worked on developing and testing a hybrid ensemble-variational (EnVar) algorithm with an extension to four dimensions that does not require the use of an adjoint model, which was implemented operationally at NCEP in 2016.
Xin-Zhong Liang is the main developer of several regional and global climate models, including the widely used regional climate model CWRF, with many new physical parameterizations. His models have been applied to study seasonal-interannual-decadal climate variability and predictability, including Monsoons, ENSO, climate feedbacks, and impact of anthropogenic emissions. Liang's research topics include: Subseasonal to seasonal climate prediction, Mesoscale regional climate model downscaling, Climate change projection and impact assessment, Regional earth system model development and application, Food-energy-water nexus and sustainability, Climate-crop interactions and agricultural productivity, and Machine learning for climate related decision support.
Takemasa Miyoshi, now Team Leader of Data Assimilation at RIKEN, Japan, where the K supercomputer is located, is interested in data assimilation with chaotic dynamical systems, particularly the weather systems and other components of the climate systems, and even the Martian atmosphere.
Steve Penny is working on ocean and coupled-model data assimilation from research to full operational implementations and fundamental methods in data assimilation. He has designed novel methods for data assimilation under non-optimal conditions, such as sparse observational data sets, short spin-up times, including the Gain Hybrid method tested with success at ECMWF.
Da-Lin Zhang works on the modeling and understanding of fundamental processes taking place in squall lines, mesoscale convective complexes, hurricanes and heavy rainstorms (or snowstorms), tropical and extratropical cyclones, gravity waves, frontal circulations and topographically generated weather phenomena. His research interests also include the development and improvement of the planetary boundary layer and cumulus parameterization techniques, cloud representations in mesoscale numerical models, and the improvement of warm-season quantitative precipitation forecasts and severe weather warnings.
Advising
Advising Faculty
- Berbery
- Carton
- Ide
- Liang
- Nigam
- Poterjoy
- Wenegrat
- Zhang
Non-Advising Faculty
- Murtugudde